21 March 2025
The Future of Work: AI's Impact on HR and Staffing with Dan Friker (266) - S7E266
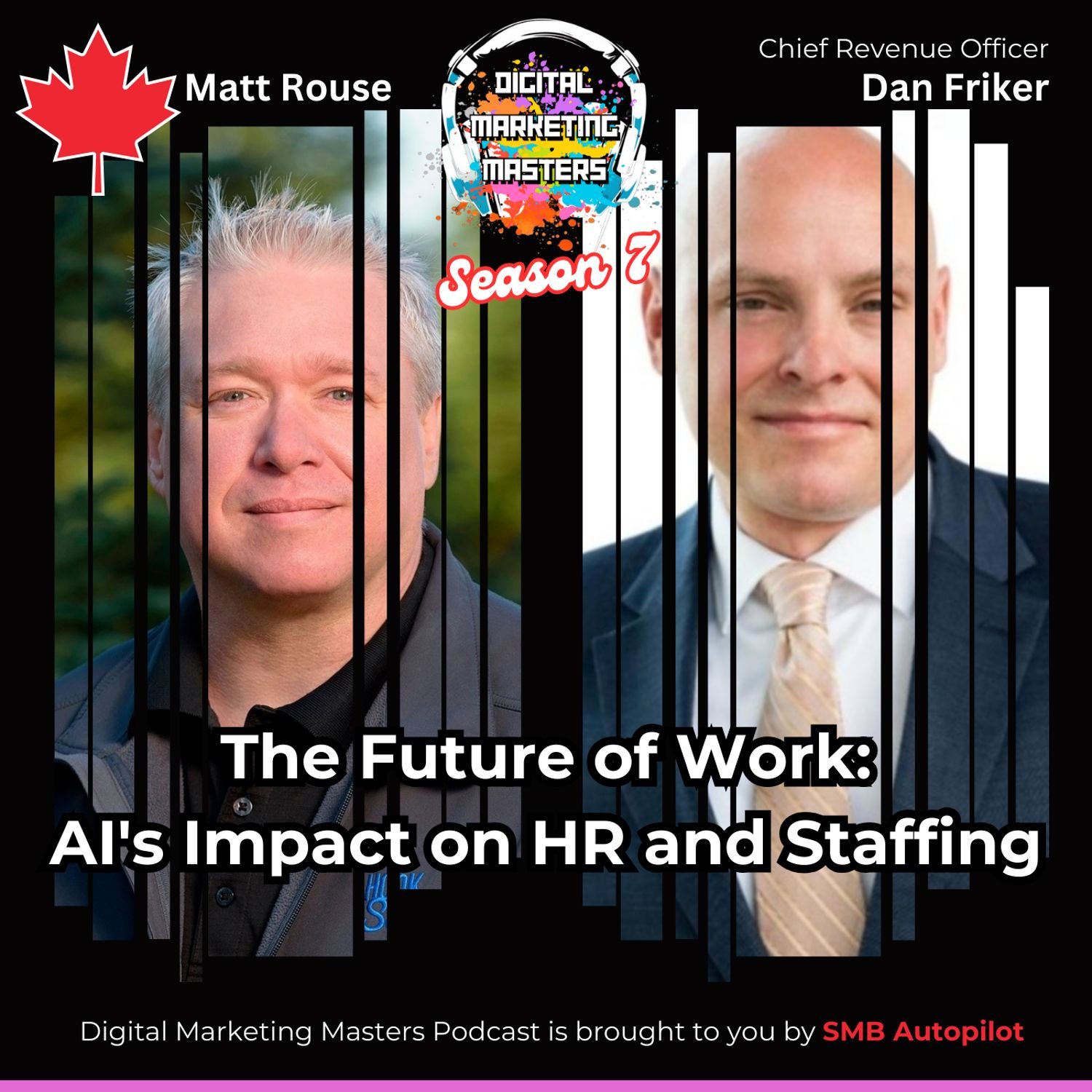
In this episode of Digital Marketing Masters, host Matt Rouse engages in a thought-provoking conversation with Dan Friker about the future of work and the impact of AI on various industries. Dan shares insights from his experience on the career advisory board for the College of Science and Engineering and discusses the implications of rising technologies on the workforce, from blue-collar to white-collar jobs. The discussion delves into how AI is transforming HR practices, including job descriptions, candidate selection, and interview processes, and the challenges of bias and efficiency in these systems.
Dan and Matt explore the global landscape, highlighting examples from countries like Japan and India, where digital literacy and AI adoption are advancing rapidly. They discuss the paradox of efficiency and success in AI-driven recruitment and the potential for AI to revolutionize staffing models in industries like healthcare and agriculture. The conversation also touches on the broader implications of AI in the workplace, emphasizing the need for workers to adapt to new tools and technologies to remain competitive in the evolving job market.
Dan Friker: https://www.linkedin.com/in/daniel-friker/
Matt Rouse: https://www.linkedin.com/in/mattmrouse/
(00:31) Introduction to the Future of Work
(01:05) Dan Fricker's Background and Passion
(02:12) AI's Impact on HR and Staffing
(05:04) Efficiency and Success Paradox in AI Hiring
(12:41) Bias and Challenges in AI Recruitment
(26:00) Blind Spots in Staffing and HR
(34:13) Future of Work and AI's Role
Today today on the digital digital marketing podcast. Your host, Matt Roose,
[00:00:15] AI Narrator #1:
talks to Dan Friker. About the future of work. Work. Work. Future of work. Future of work.
[00:00:31] Matt Rouse:
Welcome back, everybody, to Digital Marketing Masters. I'm your host, Matt Rouse. And today, I have Dan is it Friker or Fricker? I was like Fricker. Fricker. Alright. Yes. Well, I watched you on another show, and I think the guy pronounced it two different ways. I'm kinda confused with you. That's probably the with the last name of Friker, this is not the first time someone's made fun or mispronounced my last name. The thing that really caught my eye was talking about kind of digital futurism. Right? What is the future of work? So we're gonna talk about that. Do you wanna just give, like, a one breath intro to who you are, and then we can get straight into it? Sure. My name is Dan Friker.
[00:01:08] AI Narrator #1:
Undergraduate degree and a graduate degree from the University of Minnesota. I currently sit on the career advisory board for the College of Science and Engineering, so all those physicists, astrophysicists, chemists. But it has been a passion of mine around the changing world of work. And in particular, based on my kind of unique background, the implications of rising technologies on all sorts of workers from blue collar to black collar to white collar and everything in between, and and quite frankly, how not just Fortune one hundred companies, but even small, medium sized businesses are going about trying to find talent and skills. Things there are changing.
[00:01:44] Matt Rouse:
Yeah. I've been working on the new version of the book, Will AI Take My Job. So I've been also, you know, trying to take in all the new stuff as quickly as I can. You know, as actually on on the previous episode, two sixty five with Ethan Holland, he collects and organizes and summarizes all the news about AI. And in the last few years, he's collected, I think it's 23,000 AI links and summaries. So you can imagine how hard it is to keep up. Yes. Dan, I wanted to the first thing I wanted to ask you is for the show, we were talking about a bit about how AI is going to affect HR and the future of of work, not just work itself, but actually staffing. So I guess my first question is, what do you think is gonna change in HR in the short term?
[00:02:33] AI Narrator #1:
Well, I think you can see a couple of things, and you can look to certain places in the world for a little bit of insight, if you will, some little harbingers of of what's to come. Right? Right. So I was recently in Tokyo, and one of the things that I find striking straight away is when you have a country with an aging population and you have relatively still, restrictive controls in terms of how talent can actually migrate into the country, Right? You won't see anyone selling you a newspaper, assuming that someone's actually, you know, reading newspapers these days. But Right. Like, you see the amount of, like, vending machines that will sell things that will blow your mind. Right? And it's this really interesting kind of culture around there's a lot of reverence around certain things like calligraphy.
I still believe that they are the largest users of fax machines. No joke. Complete series. But yet also have some of the most sophisticated HR systems that I that I've ever seen. You also, though, have interesting harbingers when you take a look at, you know, the India and the Malaysian, the Singaporean, and even the some of the Latin American markets where you know? And studies have shown that that younger workers today are much more comfortable using mobile technologies, their their smart device, to not just search for jobs, but to actually accept jobs. Right? So, like, in the West, you know, where we, you know, we kind of evolved, you know, having things like PCs and whatnot. A lot of my Asian friends still find it hilarious that you can go to New York and still only half the restaurants or whatever have tap and pay. Like, when they see someone pull out a credit card, they laugh. They're like, oh, you still use those.
Right? So the digital literacy in certain countries, I would actually argue, are are possibly even a couple years further along than you would see, certainly The US being slightly Canada as well, because if you are now able and these are examples that I have found right now today, Right? Where people aren't just getting selected based on their mobile device app and leveraging some of these new, you know, AI, you know, recruiting tools. And then on the back end, companies are able to do a better job at not only hiring talent, but they have a way on the back end to start sorting that talent in a different way. Right? So now you're creating this kind of infinity ecosystem of talent.
And instead of doing annual reviews annually, you can now do them in an iterative way. Because in the past, if it come if you if labor up and leaves your organization, you talk about attrition. You can talk about people leaving. Now you can actually detect it before it happens. You know? Are people getting upset because of their compensation? Are they upset because of benefits? Are they upset because of shift, a manager? Now you can actually start proactively addressing these issues before people essentially vote with their feet. Right. Right? So you can see these examples that are now several years in advance, and then I look to US examples and even talk to several CHROs, and it's like they're simultaneously starting to use AI for writing, you know, job descriptions, which is great. But then you also don't you're not utilizing some of the AI tools effectively that are coming out with with some of the most common applicant tracking systems and veteran management systems that you have out there today. Right. CHRO is chief,
[00:06:12] Matt Rouse:
human resource officer. Officer. Yeah. I understand that wasn't a very common term. I when I first saw it, I tried to type it into Google. It just keeps saying Chrome. And I'm like, no. I want c h r o. It's like Chrome? Stop it, Google. I know what you're doing. Alright. So I and we had been talking about this, and I think this is an interesting idea. So the the and this is my understanding of how it works in in, let's say, medium sized corporations right now is the HR manager has previous job listings. So they take that and they put it into the AI, and then they say, this is the new differences of in in, you know, the job that we're hiring for now. So the AI is helping to write the job description.
Correct. And then they put it upload it to a job, you know, posting place or whatever it is that they're they're gonna hire through. And then that AI is sorting through the candidates and and the systems to figure out who it's gonna surface the job to to show in the first place. Correct. The peep the candidates are using the AI to write their resume.
[00:07:19] AI Narrator #1:
Percentage of them. A percentage of them. So you you you have a derivative of people starting to to write. So, again, right there, you're starting to see the have and have nots. But carry on. Fairly soon,
[00:07:30] Matt Rouse:
you know, you're gonna have people actually have the system go apply for the jobs for them. Correct. And then in the interview process, a lot of times, HR reps are using the AI to write the interview questions. Correct. And then you've got AI systems that give the person being interviewed live notes while they're being interviewed over Zoom or something. Right? It helps them come up with answers to questions live while they're on the call. Correct. And then you're using an AI system to go through all the candidate resumes and and and interview responses and everything and kind of weed out candidates. So there's a lot of AI involved in this process already.
[00:08:06] AI Narrator #1:
There's elements of AI, but there is an efficiency and success paradox. Right. And the paradox is, yes, you can have AI right now. You can LinkedIn. You can hit easy apply, and you can probably submit to hundreds of positions right now today or certainly this week. Right? That is a very efficient thing to do. Right? However, is that really the a successful outcome? LinkedIn themselves says that 95% of easy applied respond don't even get a response. Right. So it only has a five percent success rate. What is even more interesting, because I'm old, and so I re so when you look at the I'm gonna give you a quick history lesson to kind of bring you up to speed of where we are today. Right? Because the paradox is we're actually seeing entropy and efficiency of being able to link people with organizations that want to hire them.
Right? And that's doesn't make sense because they're like, wait a minute. With all these systems and with all these new versions of chat, like, we should be seeing more efficiency. And I think we will get there to be clear. But we're at this weird inflection point. Because of the nineties, you had major companies and certainly the major staffing companies, which I came from one. You know, you had these kind of traditional client server databases, and those databases were, you know, really built off of decades of people walking through a storefront and essentially human beings surveying their skills. Right? So in the nineties and early two thousands, that was actually proprietary.
You know? In fact, you would sell essentially, like, we have a better database of candidates in this market Right. With these skills versus our competitors. It was the late nineties, early '2 thousands that you saw the rise of essentially job boards, your your gen ones, monsters, career builders, that sort of thing. And that started to level the playing field in a big way. Right? Not only was it significantly cheaper, but it gave companies access to talent pools themselves versus actually, you know, going out in the marketplace and utilizing, you know, one of the big staffing companies. And what you saw was the number of placements per recruiter essentially go up.
Right? So certain, you know, white colored, you know, positions, you know, you try to benchmark, like, four placements per month, for example. There's gonna be different numbers for different types of skills. You saw those, you know, continually go up. And then they peaked because instead of finding that needle in a haystack, now you're just getting flooded with needles to find the best needle. This voice over used to be done by a human, but now it is synthetic.
[00:10:47] AI Narrator #1:
Oh la la. If you want to know if your job or business is safe from disruption, read Matt's new book, Will AI Take My Job? Predictions about AI in corporations, small business, and the workplace. Available now on Amazon.
[00:11:04] AI Narrator #1:
Trust me. It'll be worth it. Right. Right. I I think one person that explains this the best is is actually a gentleman named Tony Sultana. He wrote a great book basically, talking about why business transformations fail. Right? The example that he uses is really talking about the retail space, about how 2018 in The United States, you saw more companies go out of business. In 2018, it was it was the high watermark. Right? Right. And it was because retailers and executives at retailers like Sears, they saw the rise of ecommerce come in the nineties. They saw it in the early two thousands. But it really comes down to those that adopted new strategies and adapted those strategies inside their organization, like your Walmarts, right, that is using AI to to streamline their supply chain so that they can provide products, you know, I think five to 10,000 products within a five mile radius of a Walmart store, if I remember the Wall Street Journal article correctly, so that they can essentially do same day delivery, you know, and compete.
So you're seeing those companies adopt, adapt, and those that don't get lapped. And 2018 is when they essentially went out of business. Right? And then right now, you're seeing that with AI and HR. All these new tools that can do different little bits. So your earlier comments were completely accurate. You know? AI to write your resume, AI to write the job description, you know, AI functionality, a lot of these kind of back end HR systems. But we're not there in terms of truly linking people with positions.
[00:12:41] Matt Rouse:
Yeah. So Well, you know what I think is is another issue, and this happens in I mean, everywhere that I go talk about AI, I see the same thing. So what happens is you've got a very small number of early adopters who are very excellent at using the tools for productivity. And then you've got kind of a group of people who are kind of dabbling in it, and they're just kind of, yeah, I use it for this, I use it for that, but I don't really use it that much kind of thing. We're not getting a huge benefit out of it, but they're getting some benefit out of it. And then you've also got another small group of kind of naysayers who were like, anybody who uses this is an idiot, and AI is just a lying machine that is gonna burn all the water in the world and whatever other BS that they're gonna talk about. Right? So, like, you've got these groups of people who are are just they're either not using it or they're not using it to its its potential or they're against using it.
And that is a larger percentage of the market than the people who are using tools to their advantage. So the net advantage is is low because you have to look at it with all the other people who are not using it as part of the equation. Again, but couldn't you make the same argument in ecommerce and retail in the nineties, you know, in early two thousands? Right. But in the early two thousands, you have to remember that they started doing things like like data mining, which essentially was AI before Gen AI. Right? And before large language models, they're doing data mining and, like, SAP has a stopwatch on every single point all the way through the entire, you know, chain of the ecosystem for their supply chain all the way from, we know that there's going to be a hockey tournament in three months. So we need these jerseys to start now in Bangladesh of getting sawn and here's the color codes for Nike. Right? Like I worked on those systems. I know how they work. And a lot of that was not just retail and ecommerce colliding. A lot of it was supply chain and delivery management.
[00:14:41] AI Narrator #1:
Yeah. No. It I I'm smiling because I remember getting working on some SaaS forecast servers for a major retailer back here in Minnesota. And it was crazy about how we could take all these different variables to create, you know, staffing models for their stores based on the time of year, what Samsung was gonna have a a a new product release. And Right. You know, like, previously, this retailer was basically just allowing individual store managers to kinda staff their stores. And if that store wasn't doing as well, maybe they just kinda purposely understand it. You know? But it it was kind of that first iteration, if you will. Right? Now, Matt, I'll give you an example of something we talked about in the preshow, you know, which was health care. Right? Right.
In grad school and this is when we were trying to apply some data science to probably one of the most difficult staffing models we can do, which is staff in a hospital. Right? If you think about it, you your hospital can go out of business if and you overstaff it. Right? Right. Because, like, now now it's like you had you've you've built this In The United States, it can. Right. True. Right. Although I don't think I've seen an overstaffed hospital ever in Canada. To be But but that's the the main point is that there let's just say there's a financial incentive to make sure that the the hospital is optimally staffed. Right? And even down like, you know, even down to some simple questions of, like, you know, do you have a full time radiologist on staff, or do you essentially use an outsourced provider? Because, you know, if you're a major hospital in Downtown Minneapolis, it probably makes sense. You have enough volume to, you know, kind of handle that. But now you continue to go down that list.
And I will tell you, we were using crazy, not for the time, data science modeling to try to figure it out. And every time we were able to come close to it, how do you prepare for a major, you know, snowstorm that hits and suddenly, you know, there's more car accidents or if a plane crashes or, like, these kinda what you know, black swan events. But those are real things that hospitals have to contend with. Right. Right? AI has the potential today to create these type of dynamic staffing models so that a company if they're using AI to, let's say, create, you know, new product development, they wanna kind of pilot something new, part of the challenge you have is, okay. Do we have the talent to actually execute on that on that pilot or actually go to market?
And in in my theory is there are companies that are getting painfully close to being able to kinda connect the two.
[00:17:21] Matt Rouse:
Right? I did There's definitely some problems with connecting the dots for a lot of industries right now. Yes. They spread systems all over the place that don't talk to each other properly. And I think AI will bridge that gap. Right? I mean, right now, you can use AI with a virtual machine, open a terminal server, talk, and work its way through some system that was written in the eighties. And it's been trained on that data, so it knows how to use those systems. Right? It can use an old v t 100 terminal or something. And from the staffing system as well and and I think we saw this kind of an example of this when kind of COVID lockdowns happened, is that nobody had Slack built into the system. Everything was drilled down to as quick, as fast, as easy as we can, you know, as just in time as we can make it because that's the cheapest. And then as soon as there's any disruption, it cascades failures down all the way through the system. Right? %.
So it's like when you have a black swan event of, you know, a giant car accident because the bridge collapsed or something. Well, now you don't have anybody there because there's no slack in the system.
[00:18:26] AI Narrator #1:
And that's again, I think we're getting better at least being able to provide some of the modeling to handle some of those those talent logistics.
[00:18:36] Matt Rouse:
There could be some futuristic, you know, scenario where, you know, the AI systems are going through and they're saying, what is the chance of having an emergency based on all these other factors and all this other data that we've taken in? And it can say, well, you know, there's a lot of crumbling infrastructure around, you know, Twin Cities area, so we think it's likely that there's gonna be a problem at some point in the next six months. So we better staff more people. Unfortunately, I did live there for a while, so I know that their structure has some issues. I was in grad school with the 35 W Bridge collapsed. So I'm completely afraid. Right? You know, getting back to the HR thing, and and I think this is something I wanted to touch on again that we had talked about. I have no scientific backing for this. This is just my opinion. But if I'm a person who uses AI to write my resume for a job Mhmm.
And somebody used AI to write the job description Correct. The same correlations that happen in those systems would make it more likely for the person who applied for that job using AI to get the position by an AI checking for that thing because it's using the same correlative data.
[00:19:43] AI Narrator #1:
Right? The concept that you just talked about is actually being able to kind of see the back end, you know, like like, all the Tableau executive. So you put a job out there. You know? It's going to rank, you know, based on several, you know, algorithmic factors of, like, okay. Here are the top 10% of people that applied for this job. Right. So you're making such a I want to screen this to the to the heavens that, like, this is the implications for individuals that aren't using aren't adapting how they're going about their job search or within, you know, you know, organizations that are looking for talent, like, how they need to kind of look at this a little bit differently.
Because, yes, it's gonna give advantages to those that are using the latest AI tools because they're gonna rank really high, but you could be essentially ignoring some of the best talent because they're not using these tools.
[00:20:41] Matt Rouse:
Right. Well, now you have another problem also, which is bias starts to creep into your hiring practice based on the use of the systems. Right? So 85% of paid chat g p t users are men. Yeah. So now you've got 85% of men who have a resume that's more likely to be selected by an AI system because they wrote it with an AI system than women. So now you've got this already probably an imbalance in the men to female ratio in most industries. Right? And then you've also got this factor now that we've got more men than women using AI to apply for jobs. Right? As well as people who are generally more wealthy, first time English language speakers. Right? Languages, you know, English as a first language speakers are more likely to be using AI. There's a whole bunch of factors. People in low income areas generally don't use them. English as a second language generally don't use them. Right? So now you're not only having that specific bias creep in, but it's like a bias built on top of another bias. Right? So it's almost like exponential bias, which is terrible because you don't wanna have that happen because, obviously, you know, you're not selecting for the best candidate as you were saying.
[00:21:59] AI Narrator #1:
It it is what the EU was trying to address.
[00:22:04] Matt Rouse:
And I'm not gonna comment, is it right, is it wrong, and just like I'm not gonna comment about US But this is not this is not my opinion about DEI or something. This is a a fact of these systems. Right? It's not anything And I think politics.
[00:22:18] AI Narrator #1:
That's what again, the spirited attempt, what the EU is trying to do with the passage of their their new AI laws as it relates to HR and and hiring practices, which is number one, they want organizations to actually document how they're using AI and what are some of those preventative measures to at least mitigate bias and potential discrimination in these tools. So in other words Right. Document your process first. And then number two, like, you know, whatever those potential flaws, and then what are you doing to check to see is your system actually, you know, having unintended bias. Right? Yeah. That and you're go in my humble opinion, you're going to say that you in The US, you now have 15 states, and it will be interesting to see how many more states continue to to kind of, you know, put these similar kind of rules in place because, I mean, I don't think it's a controversial thing to say. In fact, I think it's just kind of a a standard fact that, you know, most AI models, it's all predicated on is your data you know, are you basing it off of good data? You know? And is it biased or unbiased data whether you know it or not? It it it it could show up. It does show up. So Well, I don't think there's any way to avoid bias in the scale of the data that gets entered into the systems.
[00:23:37] Matt Rouse:
Right? Because, I mean, you can't check for it because who's gonna check trillion pages of information? Like, nobody. Right? And I mean, there's lots of good data that's checked, that's human checked, and put into the systems. And I mean, they try to reduce bias post training and and and all the other ways that they do that. But I think it kind of comes back to I always think about this, I don't remember where this originally came from. But when they were having people try out to be a musician in a symphony, and they started putting the musician behind a curtain so that you couldn't see if they were male or female or black or white or whatever. Then suddenly they started hiring way more female musicians. Yeah. Because there was a bias to to thinking that male musicians were better by whoever the people who were choosing the musicians.
Right? Well, this It was the same as when they took the names off of resumes. Right?
[00:24:34] AI Narrator #1:
Correct. And I think so Andrew Nag, who was, you know, one of the founders of of Google's, you know, brain, which essentially became their, you know, AI project. Right. You know, one of the case studies that he talks about is, you know, some of those moments not in a good way was when they were saying, like, you know, man is to, you know, developer as woman is to homemaker. Like, that was the the data they were getting back. Right? And that's kind of when they started to realize, okay. There's bias here. Right. You know? I mean, the computer was just basically running the algorithm to go, okay. We're gonna go to what went over, and this is the the the value associated with that particular role. Again, what are we doing to address it? And that onus on top of a flooded inbox of employee issues is what essentially HR departments are being, you know, forced to contend with today. You know, on top of changing employment laws, on top of, you know, workforce challenges, on top of making sure that they're posting the right kind of job description based on what a department needs.
I mean, this is this is there's so many reasons why, you know, that we have inefficiency in this in this system today.
[00:25:54] Matt Rouse:
Again, I think we're gonna figure it out. It's it's the research that I need. I mean, it's difficult. Right? So let me ask you this. If in the staffing HR world, what do you think is the blind spot for most people? Like, what are they not seeing that's gonna be important now or soon?
[00:26:13] AI Narrator #1:
Oh, there's a lot. I'll give you an example. So last summer, I did a seminar. So I actually partnered with a company called Talent Edge AI. Right? And Talent Edge AI is doing some really interesting things around you know and and the CEO of Talent Edge AI is actually someone that I work very closely with when we work together at Manpower Group. Her name is Willie Gagnon. She was, let's just say, in so many respects, the right person at the right time because, you know, she was heading up a group inside of ManpowerGroup that was looking at wage data and seeing kind of how talent was migrating to different places. I mean, in real time, she was able to kind of pick up on the migration of talent, like, going from California to Texas and and whatnot and Right. Kind of using some really interesting things.
Also, taking a look at the differences in wage data. You know? So where I'm going with this is we took a so she's she knows what talent agent AI, and so we started looking at, okay. Let's examine how you take, like, light industrial workers, manufacturing workers. Like, what are the skills that they have today? And then what are the skills that companies are starting to ask for? And then let's see if we can measure that delta. Right. And when that came back, I had multiple meetings with her to go that okay. Do we do we do this wrong? Like, this can't be accurate. And, basically, what we were seeing was an exponential increase in digital skills for light industrial workers.
Right? And, initially, the data didn't make sense, but, you know, I'm I'm here in the Upper Midwest, and so it would meet with customers, meet with CHROs, meet with, you know, VPs of production. And more and more, what you're seeing is, of course, companies are buying capital equipment instead of needing 10 workers. You know, they need two because the machines are doing it all. But, of course, now they're having to work on digital screens or you know? Right. And, you know, there's there's KPIs that would come out of it in terms of production, failure rates, and everything else. There's Excel spreadsheets they have to contend with.
Well, you're essentially taking, you know, kind of minimum wage, you know, lower wage workers that now say in order to get this job, you have to have digital skills. Right. And even, like, things like around like, fundamentals around data science, you're like, an ally worker?
[00:28:42] Matt Rouse:
You know? Right. Well, I I honestly found the same thing in my own research for, you know, for the book is that the there's an edge case of workers where they need to be not necessarily the absolute best worker, but they have to be the worker who also has the ability to solve the really, really weird odd problems that come up and the skills to work with the automation that's gonna go into place. And if you have those two skills, as well as whatever the rest of the job is Mhmm. You're the one who keeps your job.
[00:29:20] AI Narrator #1:
Right? Oh, it's So Darwin did people get around all the time. Darwin thinks, oh, only the strongest survive. No. That's not what he said. That's not what he said. Adapt.
[00:29:29] AI Narrator #1:
Right.
[00:29:30] AI Narrator #1:
That's why we have the cockroach and not in brain sports works. So, you know,
[00:29:35] Matt Rouse:
again I don't know. I got some chickens, and they're like tiny dinosaurs. Yeah. You see them find a mouse or something, and you're like, man, I don't wanna be a mouse when there's some giant dinosaur chickens around.
[00:29:45] AI Narrator #1:
I mean, okay. But but, man, let's stay on something like that. Agribusiness. What so I I'm in the Upper Midwest.
[00:29:53] Matt Rouse:
Right. It's tough time to be in the bird business. I'll tell you that. Well but,
[00:29:57] AI Narrator #1:
you know, one of the biggest challenges you have is from a workforce trend perspective, you are seeing a continuous migration kind of revert a little bit during COVID, but in a sense, you know, there's less and less people in rural America or even I would even say rural Canada for that matter as well, migrated to more major metropolitan areas. And, you know, if you look at The United States, your traditional kind of tea in terms of where the vast majority of of agribusinesses are. Right? When I say tea, it's, you know, your North South Dakota, Minnesota, all the way down to, like, Kansas to, you know, Northern Texas. You know, when I started seeing at the University of Minnesota, the utilization of data science around, okay, what are those optimal crops to grow, you know Right. Within these marketplace? Well, now you have I mean, this is public now, but, like, very quietly, John Deere acquired an AI company. Right. Why? Because these guys basically found this machine to, you know, attach to the back of John Deere so that, you know, it's looking at images of weeds and applying, you know, weed killer and whatnot. So, of course, farmers could use less weed killer, optimize their their fertilizers and everything else. So now you have a bunch of automation data scientists, AI folks running around farms in ever increasing ways so that they can use less pesticides, you know, not just I mean, fertilizer costs continue to go up exponentially,
[00:31:28] Matt Rouse:
but so you're not you're you're using it more efficiently and applying it in such a way to get a better yield. Right? Right. Well, there's also, like, climate data and things like that that are getting put into those systems, and then they're automatically putting the data into the farmer's system to say, this is how much you need to water. This is, you know, this is coming up. This event might come up, you know, as well as having the equipment be able to kind of run around itself in the fields. Like I know most of the large scale farming people that I know, which is some, cause I used to live in Alberta. You know, they ride around in their tractor, basically looking at an iPad all day. You know? The tractor drives itself around the field. They don't drive it. You know? Correct. But still, when it comes time to harvest your yield, you got a small window.
[00:32:12] AI Narrator #1:
Right. And, yes, either you're going to need seasonal talent to come in or you need the truck drivers and everything else to kind of, you know I mean and and, frankly, there's less and less people, you know, in those particular areas. And, yeah, I mean, I bring this example up because I mean, Matt, you know, because of your experience in Alberta. I live here in Minnesota. So I see it. And when I have conversations with folks in San Francisco or New York, they're just, like, science and automation and farming. It's, like, more than you realize. Yeah. It's a lot. It's it's a lot. So it helps to explain the data that we're seeing in light industrial workers, but it's moving up exponentially across the value chain. And that even speaks to what that McKinsey research paper that came out in June, July of last year in terms of 75% of all workers are going to be impacted by AI, and it's gonna have different variances based on what it what kind of industry and function you're in. Right. So
[00:33:12] Matt Rouse:
I think one of the biggest differences that AI is going to be impacting kind of your middle management knowledge worker, which is different than every other kind of technology leap always affected the industrial worker. Right? It was always either the farmer or the factory worker, you know, or the laborer who was affected by a change of technology instead of, you know, the I mean, knowledge workers obviously don't have a huge history, but they are a huge swath of the economy at this point. And knowledge work is one of the places where AI systems are really good at. Right. So, you know, there is there is possible disruption there. I would say really possible, but that's just me. I I mean, we obviously, we agree on the future of work and the idea that your industrial or labor skilled workforce needs some digital skills at this point to stay competitive.
Is there anything else you think about the future of work that we should mention before we wrap up?
[00:34:13] AI Narrator #1:
I yes. And I'm going to use the war in Ukraine to help emphasize my point. Right? K. If you look at, you know, the evolution of military warfare, and I don't care how far back you go, What you see is this vicious cycle or virtuous cycle, depends on how you look at the equation, around the adoption adaption of new weapons and, of course, not just the new weapons, but the tactics associated with them. Right. Like, how do you have, you know, a country with approximately a fourth of the standing army, certainly nowhere near this, you know, the naval units and and air force units go toe to toe with a country like Russia. And the the Ukrainian war is really around kind of the first time that we've seen AI drones really level the playing field.
You know, those technologies have been around for quite some time, but it was how they actually use them to sync, for example, like the Moskva and almost completely deny the Russian navy from operating in the Black Sea. How you haven't been able to see, you know, the Russian air force essentially dominate, you know, that particular theater, and certainly we can even talk in terms of, you know, armored. That same type of practical application with these AI tools and how quickly they're coming to bear. Right? I think it's really gonna be incumbent upon organizations to kind of go through this next digital industrial revolution.
And to your point, Matt, it's gonna be incumbent upon not just your, like, industrial workers, but everyone inside the organization to be able to kind of articulate their their value and how they're incorporating these new tools to improve what they're doing or they're gonna get lapped. And I think what you're going to see in this particular new industrial revolution as it relates to AI knowledge workers is the time for them to adopt these new, you know, tools and technology is gonna be much more condensed. You know? They're not going to have years or decades to be able to adapt to these new tools and technologies because all the reports that I'm seeing are saying, okay. By 2027, by 2030, and by 2035, we're like then it's only exponentially you know, it's it's going to impact them.
So, you know, we were kinda joking before this podcast about, you know, what's coming out today with different versions of different tools. You know, probably even by the time this podcast goes out, there's probably gonna be new tools and new versions coming out as we speak. And I don't think that's gonna change. I think we're we're truly in that kind of Moore's law conundrum. It's just it's now more inclusive of all workers today. So Yeah. Honestly, I think, you know, you're right. You don't have a lot of time throughout the fax machine to start working on your, chatbot there, people, unless you're in Japan. They they still have them in Japan. I'm telling you as well.
[00:37:19] Matt Rouse:
So Yeah. I mean, I I work with a company right now called, Kanji Accelerator to help people pass the Kanji test to get work visas in Japan, which is interesting. But, yeah, it's still a requirement there. So that's that's kind of a fun job on the side. Dan, I can't thank you enough for being on the show. It's a great talk. I know we can talk forever, but, obviously, we can't. So maybe we'll have to, see if it all comes true and have you back on the show again next year.
[00:37:47] Narrator AI:
Thank you for listening to the Digital Marketing Masters podcast with your host, Matt Ralphs. If you enjoyed the show, please leave us an honest review on your favorite podcast app. Be sure to check out Matt's book, Will AI Take My Job? And have a wonderful day.
Introduction to the Future of Work
Dan Fricker's Background and Passion
AI's Impact on HR and Staffing
Efficiency and Success Paradox in AI Hiring
Bias and Challenges in AI Recruitment
Blind Spots in Staffing and HR
Future of Work and AI's Role